A list of physical infrastructure monitoring tools
Flights into Providence
https://flightaware.com/live/airport/KPVD
Shipping in Narragansett Bay
https://www.marinetraffic.com/en/ais/home/centerx:-71.5/centery:41.4/zoom:10
RI DOT Traffic Camera
https://www.mapquest.com/?center=41.809012,-71.40841&zoom=12&layer=traffic
Gritters in Scotland
http://scotgov.maps.arcgis.com/apps/webappviewer/index.html?id=2de764a9303848ffb9a4cac0bd0b1aab
Macroservices, a middle ground between the monolith and microservices.
A composition is nothing more than a declaration of a set of components to activate, their interdependencies, and their configurations. Configurations are generally properties, ie name and value pairs, accessed from the environment. Much as when you build the executable and draw all the benefits of type checking, unit testing, static analysis, etc you can build the composition and draw similar correctness assurances.
A macroservice allows your small team of developers to focus on what matters to your business, ie what your customers value. The development infrastructure is simple. The deployment infrastructure is flexible. Troubleshooting is comprehensible.
Written in response to Microservices are for companies with 500+ engineers.
Update: Perhaps a better name is "polyservices".
Update: I am reading Sam Newman's new book Monolith to Microservices: Evolutionary Patterns to Transform Your Monolith. My macroservice is more akin to his distributed monolith except that the services contained within do have stronger data independence than is typical of a monolith.
RI's Innovation Vouchers to help fund product development
I recently learned about RI's Innovation Voucher program. The vouchers are $5,000-$50,000 grants for small companies to buy the expertise needed to develop a new product or process. Vouchers can be redeemed for services at a research institution or to fund an in-house research & development project.
More information at RI Commerce Innovation Incentives.
Lucene, shadow query classes, and the visitor pattern
Defining the schema for the index is mostly an upfront design task: What are the fields? What fields are stored and what are indexed? How are terms in fields parsed? Are found terms supplemented? Are multiple fields combined? Etc.
Once you have made these decisions revisiting them can be prohibitive without planning. Ie, reindexing is found to be too expensive in time or resources, or, worse, the source documents are no longer available. Most Lucene uses error on the side of having a cautious schema where a lot more is stored and indexed than is needed now with the hope of a successful schema refactoring in the future. My experience has been that you don't know enough now about your data, how to index it, how to query it, and how users want to access it to have a viable cautious schema. It is better to store all your sources so you can reindex when you know better how the indexes and queries can cooperate. This is just a cost to using a new technology (and, as always, it can be mitigated).
The upshot in the near term is that the index schema is static. You have to apply flexibility with the query.
As mentioned in a previous posting, there is a tendency to think of Lucene queries like SQL queries. That is, there is a single, correct rendition. Discard that thinking. There are no correct results; there are only better results. To achieve better results you need to watch what your users searches and workout how the queries need to adjust. For example, perhaps you discover that there is a shift in vocabulary happening. What was once "OS X" is now "macOS". When a user queries for OS X you need to also include macOS in the query.
The Lucene API contains a number of query subtypes. These are combined to construct an expression that characterizes the user's search intent. This nested data structure should be considered a starting point. This data structure will be augmented and reformed to reflect your current understanding of how to best use the indexes. In the example above, you want to include macOS when OS X is used.
The Lucene API query subclasses are too rigid for direct augmentation and reforming. In the past the API was downright unbending and so I developed a set of shadow query classes that were amenable for use with the Visitor pattern. For example, this shows two visitors, the first adds the macOS variant and the second converts the query to a Solr expression:
Query q = ... new TermQuery(10.0f, "f", "osx") ... ... Map<String, List<String>> variants = new HashMap<>(); variants.put("a", Arrays.asList("osx, "macos")); VariantsQueryVistor vistor = new VariantsQueryVistor(0.0001f, variants); ... q = vistor.visitQuery(q); ... s = new SolrLuceneQueryVistor().visitQuery(q).toString();and s is
( "osx" OR "macos" ^ 0.0001 ) ^ 10.0
Git and the busy development shop
I don't like Git and this posting is not going to change anyone's mind about Git and I will continue to use Git as it has become the one version control system to rule them all.
Git does not work the way I want a version control tool to work and especially in a busy development shop. I work on multiple branches concurrently. Does anyone have serialized bug and feature work? I don't need the whole version control tree available all the time. I only need the trunk and the branches I am working on and I need them to be available simultaneously. Git's stash, which allows pausing development on one branch to work on another, is useless to me. Using it breaks my work flow and destabilizes my mental model of my file system. That is, when I am in ~/src/foo/branches/issue1/
I know everything below is work being done on issue1, and when I am in ~/src/foo/branches/feature2/
I know everything below is work being done on feature2. There is never any confusion as to what I am looking at. With Git, however, I need to frequently confirm that ~/src/foo/
is currently checked-out for issue1 or feature2. (Cloning to specific issue1 and feature2 directories is not a solution. See following.)
The other issue with Git is with centralized repos. Git's development model assumes many developers, each with their own full copy of the repo, and exchanging updates via patches. I understand this model and see its value. Introducing a centralized repo into this model adds complexity and collateral problems. It is not that the problems are unique to Git, but that a centralized Git repo intensives them. For example, developers A and B work on the same branch and each performs one or more checkins and some number of pushes to the origin (ie, the centralized repo). Since we have 3 repos in play -- A's repo, Bs repo, and the centralized repo -- the chances of a conflict after checkin are very high. Compare this with Subversion where there is only one repo. A and B can work as much as they like and will be stopped to resolve conflicts at checkin and not afterwards. I argue that resolving conflicts at checkin is a far better time to do this work as one's mind is in "differences" mode. When using Git I find myself performing a checkin immediately followed by a push so as to avoid this mess. Doing so loses a key feature of Git, ie distributed version control that works offline.
Update: When I wrote the following I was not aware of interactive rebase. An interactive rebase is used to reorder and combine commits so as to provide a list of changes where each change is functionally complete and the list is in a logical order. The video Interactive git rebase for code reviews and profit is a useful guide to doing that.
I hate Git's rebasing. File A is branched from master. A is changed N times on the branch and a few times on the master. Rebasing A is the equivalent of branching A from the current master and automatically applying the N changes. Doing this ruins the version history and reviewing the version history is the first step in fixing regressions. Other version history fraud comes from deleting branches and so loosing detail about who and when a change was introduced to the branch which are details that are often critical to recontextualizing the "fix". Git can be used without recourse to rebasing and deleting branches, but it seems to be common practice.
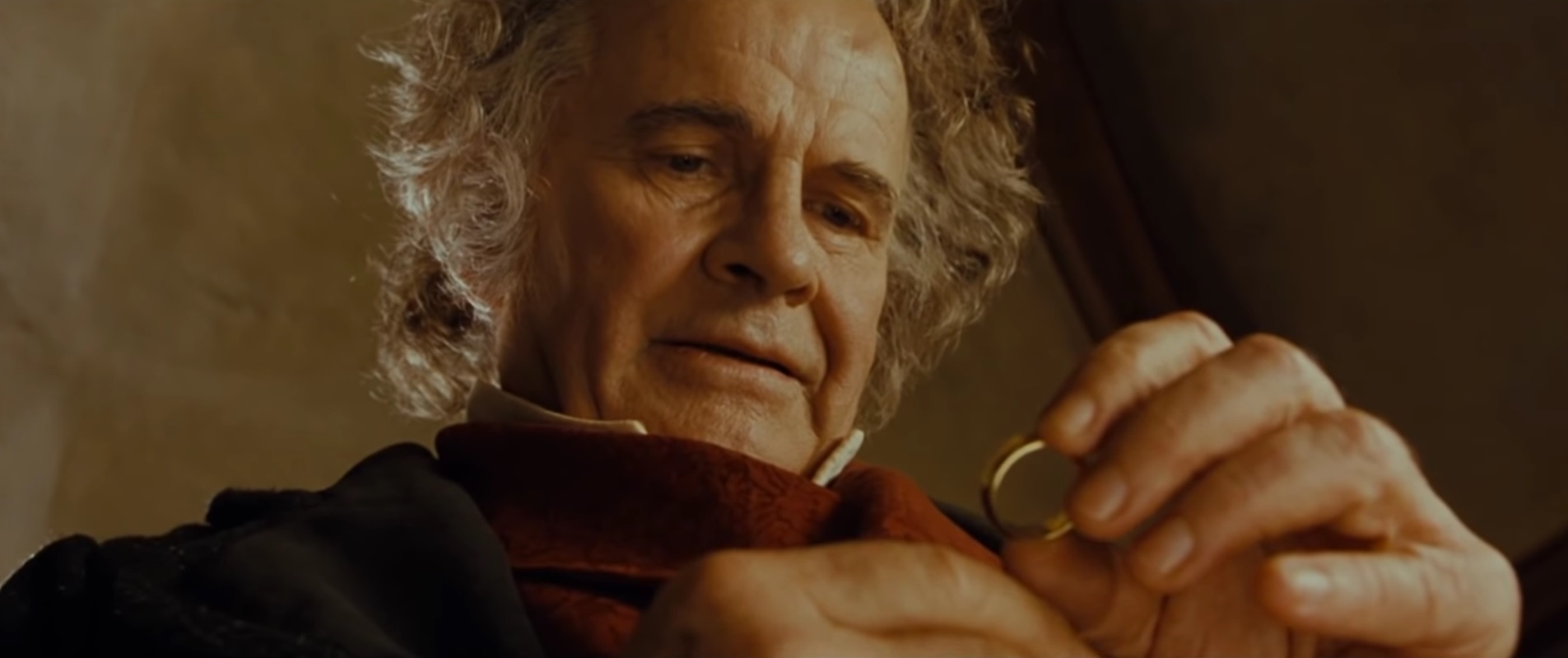
Back to coding...
Remain in control of your search
Lucene, Solr, and Elasticsearch have a powerful query language and a convenient textual representation. I have seen some API providers allow users to directly use this representation within their search API. Unfortunately, when you do so you loose significant control over your development and operations. You have exposed how you process your source data for full-text searching and so can no longer make behind-the-scenes schema changes. All your queryable data must be indexed in Lucene even if doing so is questionable and/or searchable elsewhere. You are locked into using Lucene to execute the query and at scale this can be exorbitantly expensive. To fix any of these issues you end up having to break backwards compatibility. How often will your API users accept this?
Instead, design your own query language. You can base it on Lucene's syntax if you like or an S-expression as it is trivial to parse in any programming language. No matter how you express the syntax, however, you have control over the semantics and the execution. For example, perhaps one of the searchable fields is not in your full-text index, but in a relational database and its presence in the query signals a programmatic join of the indexed and relational results. You can do that. Even if your queries are easily handled by Lucene at scale you are still better off translating yours syntax, like this old-school Google query
-a +b c d
to the Lucene equivalent
not a and ( b and (c d) )
because you remain in control.
Update: I fixed the term transposition in the Lucene equivalent of the Google expression. Doh!
Lucene boosting
Lucene has a powerful query language. The same language is used by Solr and Elasticsearch. I have found that users often don't utilize it well as they mistakenly apply their SQL experiences to it. With SQL your queries return exact results. Nothing in the result set is irrelevant. SQL query performance rewards tight queries consisting of few terms, few indexes, and few joins. With Lucene yours queries return ranked results. Much in the result set is actually peripheral. In fact, unless you limit the result set Lucene will return all the documents. A Lucene query is about getting a good ranking of results rather than exact results. To this end your query and indexes need to work together to achieve this. In general this means you need to make good use of broadening and narrowing terms, and boosting matches.
For example, if your query simply looked for the term "mouse" and you indexed your documents verbatim you should not expect to find any "mice". (Recall that Lucene sees your words as numbers and so "mouse" might be #23 and "mice" might be #6078.) It is therefore better to search for
mouse mice
When searching for "mouse mice" your results will be ordered so that either term gives equal weight to the document's rank. This is unlikely the correct course. The query was for "mouse" and you broadened it to include "mice". Documents matching "mouse" should be ranked higher than documents matching "mice". In Lucene's query language you do this by boosting the weight of terms. Documents matching "mouse" should be boosted orders of magnitude higher than "mice", ie
mouse^1000 mice
You will often see small boost values in other peoples examples. My experience has been that small boosts do not adequately differentiate documents. Big boosts do.
You likely noticed that my query was for "mouse mice" and not "mouse or mice". With Lucene, as with SQL, a boolean "or" kills performance. By not using "or" in your Lucene query you are allowing it to rank higher documents that contain both terms over documents that contain only one of them. Since the higher documents do contain the wanted term, "mouse", I don't see a need to repress ranking them equally to documents containing only "mouse" (ie, no use of "mice"). The performance cost is usually not worth it, especially when your queriers will be more complex than this simple example.
South Kingstown's impenetrable budget documents
The budgeting phrase "structural deficit" is a euphemism for spending more than you earn or spending less than necessary. That is, avoiding spending on maintenance or other costs that can be deferred or refinanced. The South Kingstown School District (SD) has clearly been running structural deficits. Implementing programs it can not afford. Delaying maintenance until unavoidable. And that this has been going on for years shows that the School Committee has failed us and the Town Council has inadequately performed their budgeting oversight.
The SD has a long history of providing impenetrable budget documents. Often the documents consist of a few printed spreadsheets and a mountain of slide decks. Both of which are incomprehensible without the oral narrative given at workshop meetings. Further, when you ask for the actual data you get files of account and values without any description of structure or purpose. This situation is unacceptable.
Not until the SD can provide an intelligible budget document that can be understood by us at our kitchen table should they be authorized to bond for any monies. Creating such a document is not a light undertaking, but luckily the SD can follow the exemplars found at Association of School Business Officials International (ASBO) and the Government Finance Officers Association (GFOA).